Apple's internal 'Overton' AI tool helps with Siri's development
Apple has offered details of an internal development tool titled "Overton," a system for monitoring and improving machine learning applications such as how Siri determines results for queries, by handling the lower-level tasks and allowing engineers to focus more on higher-level concepts.
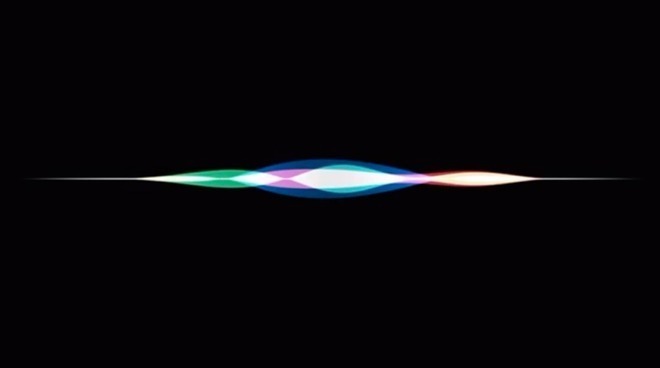
Artificial intelligence and machine learning can be a hard field to manage, working to allow systems to perfectly understand statements, to recognize an image, or to help power self-driving vehicle systems like Apple's "Project Titan." The problem with machine learning development is that engineers have to closely examine how the data is parsed, and to determine how exceptions to normal data should be managed, a task that is only going to get harder as systems get larger and more sophisticated.
To that end, Apple produced the "Overton" framework, a research paper by Apple engineers spotted by VentureBeat advises. Overton is designed to automate the training of AI systems by offering high-level abstracts provided to it by engineers.
For example, Overton could generate a model to supply the answer to a question that may be tricky for digital assistants like Siri to parse, such as "How tall is the President of the United States?" This sort of query requires multiple data pipelines to be sourced, with many parts to ascertain before creating the intended answer.
Normally engineers would spend most of their time working on fine-grained quality monitoring of unusual data subsets, as well as supporting said multi-component pipelines. With Overton, Apple intends to limit the amount of work an engineer needs to do, automating many of the chores and to keep monitoring elements on behalf of the engineers.
"The vision is to shift developers to higher-level tasks instead of lower-level machine learning tasks," the paper states. "Overton can automate many of the traditional modeling choices, including deep learning architecture, and it allows engineers to build, maintain, and monitor their application by manipulating data files.
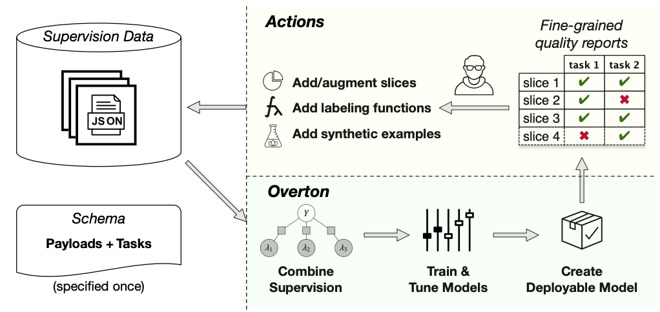
A high-level illustration of Overton's features
Furthermore, Overton is produced in such a way that it could be interacted with "without writing any code." Instead, Overton creates a schema from data payloads that describe input data used for AI model training, as well as model tasks that describe what the model needs to perform.
The schema also defines the input, output, and data flow of the intended model, with Overton compiling it into a variety of AI development frameworks, including TensorFlow, CoreML, and PyTorch, to determine the most appropriate architecture for model learning.
Overton is also able to use techniques like model slicing to identify subsets and reduce bias, as well as multi-task learning to predict all of a tasks a model may require.
So far, Overton has been valuable to Apple's researchers, with errors reduced between 1.7 times to 2.9 times against production systems.
"In summary, Overton represents a first-of-its-kind machine-learning lifecycle management system that has a focus on monitoring and improving application quality," the paper reads. "A key idea is to separate the model and data, which is enabled by a code-free approach to deep learning."
Apple's machine learning work is considerable with a growing workforce and knowledge base via various acquisitions, and touches many different areas of its software business. Most notable is its work with Siri, but the results of its research also surfaces in other elements, such as iOS 13's ability to detect cats and dogs in photographs.
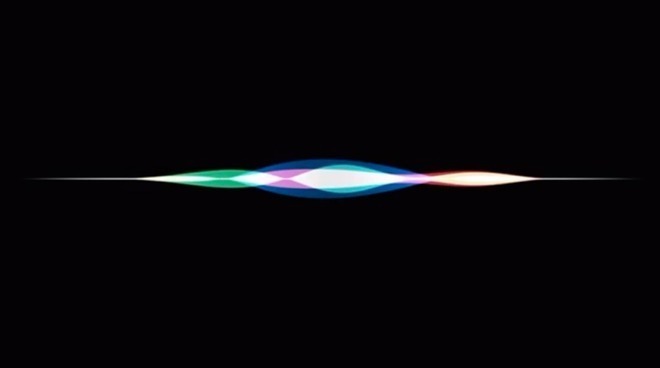
Artificial intelligence and machine learning can be a hard field to manage, working to allow systems to perfectly understand statements, to recognize an image, or to help power self-driving vehicle systems like Apple's "Project Titan." The problem with machine learning development is that engineers have to closely examine how the data is parsed, and to determine how exceptions to normal data should be managed, a task that is only going to get harder as systems get larger and more sophisticated.
To that end, Apple produced the "Overton" framework, a research paper by Apple engineers spotted by VentureBeat advises. Overton is designed to automate the training of AI systems by offering high-level abstracts provided to it by engineers.
For example, Overton could generate a model to supply the answer to a question that may be tricky for digital assistants like Siri to parse, such as "How tall is the President of the United States?" This sort of query requires multiple data pipelines to be sourced, with many parts to ascertain before creating the intended answer.
Normally engineers would spend most of their time working on fine-grained quality monitoring of unusual data subsets, as well as supporting said multi-component pipelines. With Overton, Apple intends to limit the amount of work an engineer needs to do, automating many of the chores and to keep monitoring elements on behalf of the engineers.
"The vision is to shift developers to higher-level tasks instead of lower-level machine learning tasks," the paper states. "Overton can automate many of the traditional modeling choices, including deep learning architecture, and it allows engineers to build, maintain, and monitor their application by manipulating data files.
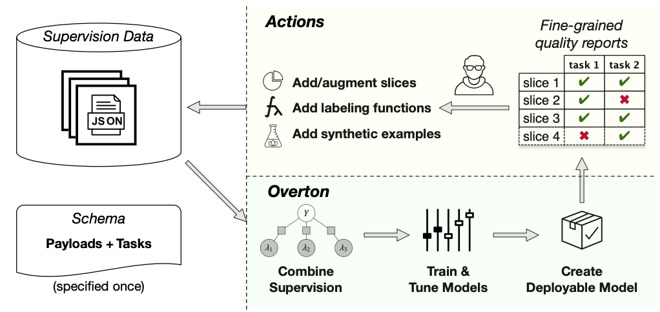
A high-level illustration of Overton's features
Furthermore, Overton is produced in such a way that it could be interacted with "without writing any code." Instead, Overton creates a schema from data payloads that describe input data used for AI model training, as well as model tasks that describe what the model needs to perform.
The schema also defines the input, output, and data flow of the intended model, with Overton compiling it into a variety of AI development frameworks, including TensorFlow, CoreML, and PyTorch, to determine the most appropriate architecture for model learning.
Overton is also able to use techniques like model slicing to identify subsets and reduce bias, as well as multi-task learning to predict all of a tasks a model may require.
So far, Overton has been valuable to Apple's researchers, with errors reduced between 1.7 times to 2.9 times against production systems.
"In summary, Overton represents a first-of-its-kind machine-learning lifecycle management system that has a focus on monitoring and improving application quality," the paper reads. "A key idea is to separate the model and data, which is enabled by a code-free approach to deep learning."
Apple's machine learning work is considerable with a growing workforce and knowledge base via various acquisitions, and touches many different areas of its software business. Most notable is its work with Siri, but the results of its research also surfaces in other elements, such as iOS 13's ability to detect cats and dogs in photographs.
Comments
https://en.m.wikipedia.org/wiki/Overton_window
Maybe something more innocuous? Like SkyNet, perhaps.
I am Apple fanboy, but SIRI just sucks.
Siri and the Siri knockoffs all suck.
The difference is people only get emotional with Apple products which spawns the "Siri sucks!!" comments.
It’s mostly a better understanding of context for tasks around what the operating system should support through finger gesture interaction anyway, a reduced failure rate in understanding and recognition of my voice in a way that approaches faceID (because if Siri can do stuff like send a document I’ve stored to someone around afternoon, Siri shouldn’t listen to my colleague requesting this from my phone).
With context I mean: where am I? What am I doing? What was I working on? Where am I going? And then interpret my query around factors like these.
Lastly, Siri should support ‘default apps’, e.g:
- default maps app
- default music app
- default browser
- etc
...And then when I perform a Siri query, I should be able to omit Safari, Chrome or Spotify in my request (note: Siri doesn’t support most of these anyway because Apple isn’t fully opening up to competitors like Spotify).
It’s anti-competitive behavior from Apple when I have to say “give directions to home using Waze” versus “give directions to home” (which defaults to Apple maps).
Apple should be sued for that behavior.
and definitely should recognise the set default app.
me. I wonder what is google assistant is using?
Even a crude oral tag inside a query would be of help:
"Hey Siri, When did [open Spanish] Los Héroes del Silencio [close Spanish] split up?"
However I'm very interested to learn that you aren't having the same problems as me. Can I ask what your native language is?
In my case (northern Spain) I constantly run into problems between English, Catalan and Spanish.
Siri is set to Spanish but towns and street names are often only in Calatlan. For cultural references all three languages can mix in the query and that's where it all falls apart for me and I have to type things in manually.
Some instances may have a Spanish version but it is never used (not even by Spanish native speakers) as the regional word or pronunciation is seen as the correct term.
Another example is my Nvidia Shield (this time with Google Assistant). Actors' names, song titles, film titles etc. The queries could all have a mix of languages.
Still, it is interesting to learn you aren't having such a hard time in this area. I wonder how many bi or trilingual members here are doing with their respective languages when mixing languages within the query.